Artificial Intelligence – Technology for Special Assignments
Artificial Intelligence (AI) has become more than just a buzzword in recent years. With its ability to simulate human intelligence and automate complex tasks, AI has emerged as a powerful technology for special assignments. Leveraging AI can help businesses streamline their operations, enhance decision-making processes, and unlock new opportunities. While AI can be implemented both on-premises and in the cloud, cloud-based AI services offer several advantages such as scalability, cost-effectiveness, and easy integration with existing systems.
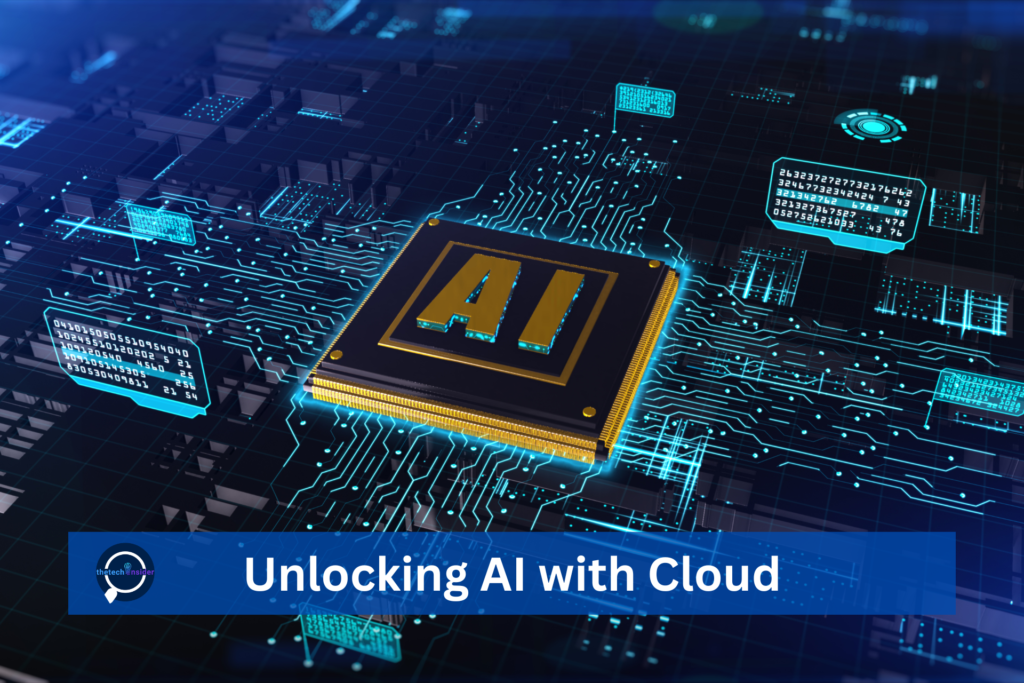
Benefits of Cloud-Based AI
- Scalability: Cloud-based AI services provide businesses with the flexibility to scale their AI capabilities as per their requirements. The cloud infrastructure allows for the seamless allocation of computational resources, ensuring that businesses can leverage AI power without worrying about hardware limitations.
- Scalability enables businesses to accommodate varying workloads and handle peak demand periods efficiently.
- With cloud-based AI, enterprises can rapidly deploy and test AI models, facilitating quicker time-to-market.
- Cost-Effectiveness: Cloud-based AI services eliminate the need for significant upfront investments in hardware and infrastructure. By adopting a pay-as-you-go model, businesses can optimize their AI investments and avoid unnecessary expenses.
- Cloud-based AI reduces maintenance costs associated with managing on-premises infrastructure and allows businesses to focus on core competencies.
- Integration and Collaboration: Cloud-based AI services seamlessly integrate with existing organizational systems and technologies, enabling businesses to leverage their investments and avoid the complexities of building infrastructure from scratch.
- Integration with existing systems promotes collaboration between different departments, fostering a unified approach to leveraging AI technologies.
Real-Case Scenarios of Cloud-Supported Artificial Intelligence /Machine Learning
Cloud-supported AI and Machine Learning (ML) are transforming various industries across the globe. Here are a few real-case scenarios that demonstrate the power of cloud-based AI/ML:
- Healthcare: Cloud-based AI is revolutionizing the healthcare industry by enabling accurate diagnosis, personalized treatment plans, and efficient patient management. With the ability to process vast amounts of medical data, AI algorithms can identify patterns, predict outcomes, and assist healthcare professionals in making informed decisions.
- Cloud-supported AI models can analyze medical images, detect abnormalities, and enhance diagnostic accuracy, leading to improved patient outcomes.
- ML algorithms deployed on the cloud can analyze patient data to identify high-risk individuals and recommend preventive measures, transforming healthcare from reactive to proactive.
- Retail: Cloud-based AI empowers retailers to enhance customer experiences, optimize inventory management, and gain valuable insights for targeted marketing campaigns. By analyzing customer behavior and preferences, AI algorithms can personalize product recommendations, driving customer satisfaction and loyalty.
- AI-powered chatbots integrated with cloud-based services can offer personalized customer support, improving response times and reducing operational costs.
- ML models coupled with cloud infrastructure can analyze sales data and predict demand, enabling retailers to optimize their inventory levels, reduce wastage, and increase profitability.
- Finance: Cloud-supported AI/ML is reshaping the finance industry by automating risk assessment, fraud detection, and portfolio management. AI algorithms can analyze vast financial datasets, identify patterns, and make accurate predictions to enhance decision-making.
- Cloud-based AI models can detect fraudulent transactions in real-time, protecting financial institutions and customers from financial crimes.
- ML algorithms deployed on the cloud can assist in portfolio optimization, providing investors with tailored investment strategies and improving portfolio performance.
MLOps – Machine Learning Model Management
As businesses embrace cloud-supported AI/ML, the need for effective machine learning model management, commonly known as MLOps, arises. MLOps represents the combination of machine learning practices and DevOps methodologies, designed to streamline the deployment, monitoring, and management of AI/ML models.
Key components of MLOps include:
- Version Control: MLOps emphasizes the importance of version control to track changes made to AI/ML models over time. Version control enables reproducibility, transparency, and collaboration among data scientists and engineers.
- Continuous Integration/Continuous Delivery (CI/CD): CI/CD practices ensure the seamless integration and deployment of AI/ML models into production environments. By automating the testing, building, and deployment processes, organizations can minimize errors and accelerate time-to-production.
- Monitoring and Maintenance: MLOps incorporates monitoring tools to continuously evaluate model performance, detect anomalies, and identify the need for model retraining or updates. Proper monitoring enables organizations to ensure the reliability and accuracy of AI/ML models throughout their lifecycle.
AI/ML Services Will Lead the Way for Businesses in 2023
The adoption of cloud-supported AI/ML services is poised to accelerate in the coming years, with businesses recognizing the significant value and potential they offer. According to industry experts, AI/ML services will be at the forefront of transformative technologies for businesses by 2023.
Key reasons why AI/ML services will lead the way for businesses in 2023:
- Competitive Advantage: Implementing AI/ML services allows businesses to gain a competitive edge by leveraging data-driven insights, automating processes, and delivering personalized experiences to customers.
- Innovation and Agility: Cloud-supported AI/ML services offer businesses the flexibility to experiment, innovate, and adapt to changing market dynamics swiftly. The ability to rapidly develop and deploy AI/ML models fosters a culture of innovation within organizations.
- Cost Savings: Adopting cloud-based AI/ML services eliminates the need for significant upfront investments in infrastructure and provides cost savings in terms of maintenance, scalability, and operational costs.
Conclusion
In conclusion, embracing the power of cloud-based services opens doors to leveraging artificial intelligence. Cloud-supported AI/ML provides businesses with scalability, cost-effectiveness, and easy integration, revolutionizing industries such as healthcare, retail, and finance. MLOps plays a crucial role in managing AI/ML models effectively. Looking ahead, AI/ML services will continue to lead the way for businesses, offering competitive advantages, driving innovation, and delivering cost savings. Embrace the limitless possibilities Al-powered cloud services bring and unlock the full potential of your business.
Frequently Asked Questions (FAQs)
Q: What are the key benefits of using cloud-based AI services?
A: The main benefits are scalability, cost-effectiveness, easy integration, faster innovation, and avoiding upfront infrastructure costs. Cloud-based AI can scale flexibly with demand.
Q: How can cloud-based AI help the healthcare industry?
A: In healthcare, AI on the cloud can enhance diagnosis through medical image analysis, predict outcomes, optimize treatment plans, and improve overall efficiency.
Q: What is MLOps and why does it matter?
A: MLOps focuses on effectively managing machine learning models via version control, CI/CD, monitoring, and maintenance. This improves reliability and lifecycle management.
Q: Why will AI/ML services lead businesses in 2023?
A: AI/ML provides a competitive edge through data insights, innovation, adaptable systems, and cost savings – key advantages for businesses.
Q: What risks exist when adopting cloud-based AI?
A: Potential risks include data security if not properly managed, over-reliance on black box models, and integration challenges with complex legacy systems.